Gone are the days when retailers could afford to build forecasting models based on some historical sales and demographics that would be updated every couple of years.
What 2020 has demonstrated to many retailers is that in a matter of weeks, demand for certain products can skyrocket instantaneously. Sales capture can shift from purely in-store to online by tenfold. Civil unrest and injustice can scar the customer experience for brands without solid social, environmental, and corporate governance policies.
The efforts that teams put into market planning can unravel overnight, and the strategies left in place are only as resilient as the datasets and tooling afforded to them. No longer can static visualizations based on historical, and sparse datasets be relied upon to drive sustainable network performance.
With constantly changing consumer markets, dynamic datasets and analytical modelling that reflect the consumer’s ever-changing reality need to be leveraged in order to adapt and plan during uncertain times.
“‘Understanding your customers’ tends to be an aphorism that is thrown about in business contexts,” explains Lyden Foust, CEO of
Spatial.ai.
“Historically, it has been fuzzy, hard to measure, and at best, purely anecdotal. But during periods of economic uncertainty, ‘understanding your customer’ is no longer a nice to have. Luckily, with the datasets that exist today, this fuzzy concept has transformed into a quantitative discipline that will be crucial as we move into intelligent recovery.”
Spatial.ai organizes billions of public, geotagged social posts into 72 segments to reveal the customer attributes that predict product, retail, and brand success at the block group level.
Mobile location data is another example of data that gives retailers insight into human behaviour by looking at movement patterns. Movement patterns captured in mobile location data include visitor counts to a location, the types of people those visitors represent, and pedestrian and vehicle counts along road segments. Looking at this data during the pandemic demonstrated the foot traffic impact of temporary store closures. Conversely, mobile data also highlighted brand recovery as brick and mortar stores reopened (1).
Retailers leveraging adaptive datasets like Spatial.ai’s Geosocial segmentation data and Safegraph’s Mobile Location Data, alongside their standard toolbox of demographics, sales data, and competition, can better predict how to optimize their network performance in changing times.
Given the transmissibility of COVID-19, socially active retail areas have been hit harder than less socially active areas. Using Spatial.ai’s geosocial factors, retailers can monitor how social behaviors related to people getting together in groups will decrease in the face of a lockdown, whereas social behaviors that people can either do individually or while physically distancing stand to increase.
If your brand's location is in the midst of, or benefits from more of the "extroverted" variables, this does not bode well for business. Obvious examples of this include movie theaters or bars. More specifically, areas whose retail benefit from proximity to "Party Life, Girls Night Out, or Live Experiences" behaviors have been significantly negatively impacted.
On the other hand, if your brand benefits from less "extroverted" variables, this can actually be an advantage. We can point to a prime example of this by observing the huge lift in sales seen at hardware stores like Lowes or Home Depot in response to an increase in DIY activity during the pandemic. Similarly, retailers that benefit from proximity to "Outdoor Adventures, Dog Lovers, or Farm Culture" have actually seen lift in business during these uncertain times.
Through public studies, Geosocial has identified real-time changes in an area during the Covid-19 pandemic, such as one in which researchers discovered that large metros had a huge increase in "dog lover" activity during stay at home orders - which also happened to coincide with online sales for the pet eCommerce site, Chewy.com. These Geosocial fluctuations can be seen in the graphs below:
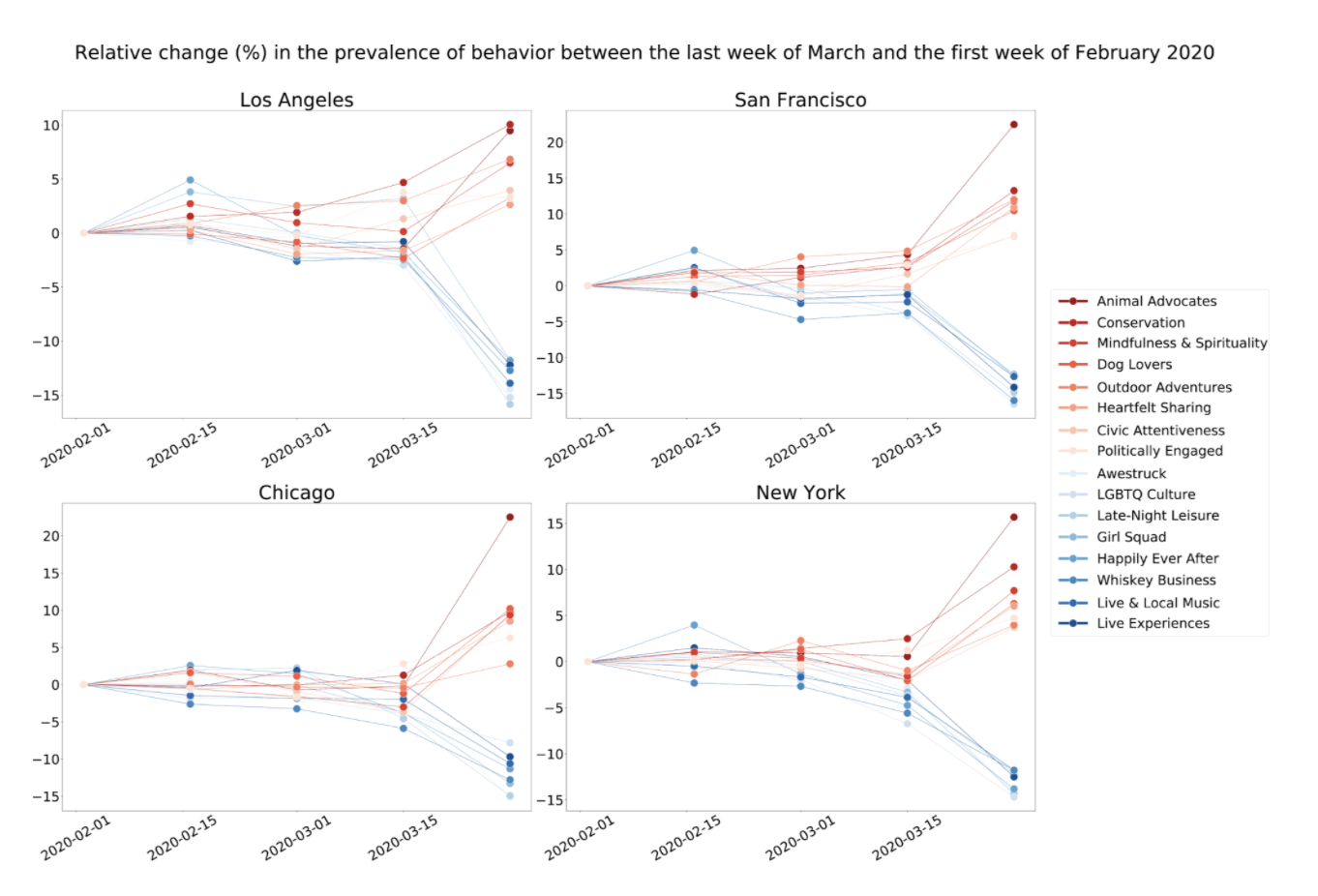
This strategy is very location and brand dependent. Some brands using Spatial.ai data have been able to detect variables that are relevant to their predictive model going down during COVID-19, such as "live experiences".
Tapping into Geosocial behaviour helps inform brand site strategy in a post-COVID world, ranging from how to consolidate existing stores to where to find new locations that represent your post-COVID customer. For example, Geosocial data can assist in identifying locations with relevant variables that actually increase during the pandemic, such as Dog Lovers, Outdoor Adventures, and Mindfulness & Spirituality.
While navigating the uncertainty of COVID-19 lockdowns and store re-openings, the more variables and factors a retailer is considering in their analytics, the less fragile their overall strategy is and the better they can predict their network’s response to change.
Great modelling can only go so far with limited or outdated data. Foust describes how demographics alone miss a critical part of the store’s indicators for success; mindsets, interests, and attitudes of the people in the catchment area since neighborhoods are fluid and demographics tend to lag.
Because Spatial.ai’s Geosocial data captures a multivariate picture of neighborhoods, retailers can differentiate a winning location from a losing location - even when both are the same using demographics.
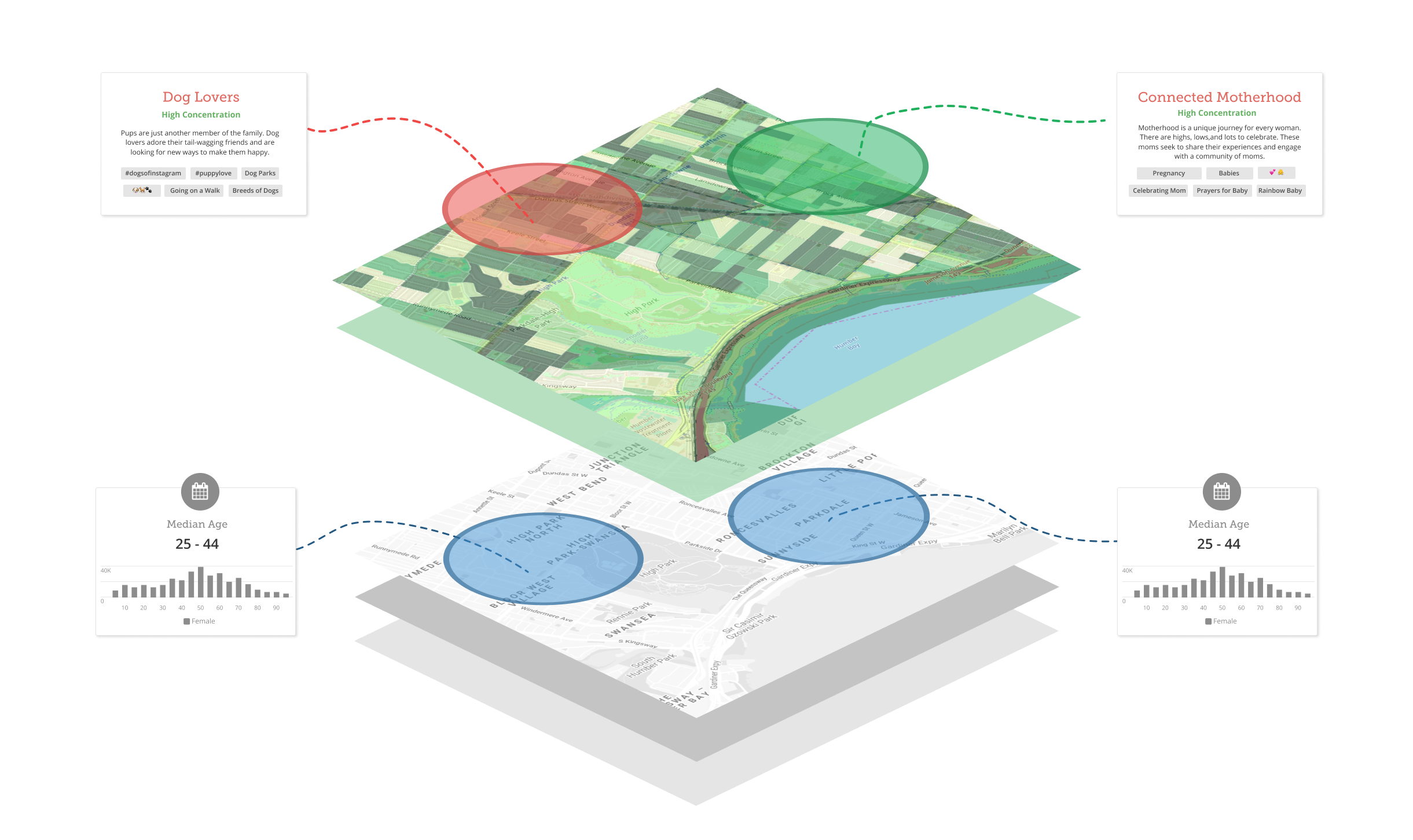
These insights are applied directly in individual scenario planning, but also as an input in predictive models.
A trend long recognized as being foundational to retail analytics this century is the adoption of machine learning (ML) technology and artificial intelligence (AI). The benefits of cutting edge tech like ML and AI are their ability to be constantly learning, iterating, and improving. Combined with datasets like mobile location data and Geosocial, which are simultaneously evolving and up-to-date, come powerful statistical models with an edge against uncertainty.
With real estate analytics, the selling point has often been that access to data and analytics takes the guess-work out of site selection, store closures, operating strategy, and so on. We agree with this statement. In fact, in these times it is perhaps more important than ever.
However, the usability and imagination of those proven models must evolve too, offering tools that extend beyond traditional concepts of growth and development. Professionals in retail uncertainty need strategies built on efficiency, longevity, creativity, and insight.